Environmental Intelligence: AI, Truth, and the Environmental Public
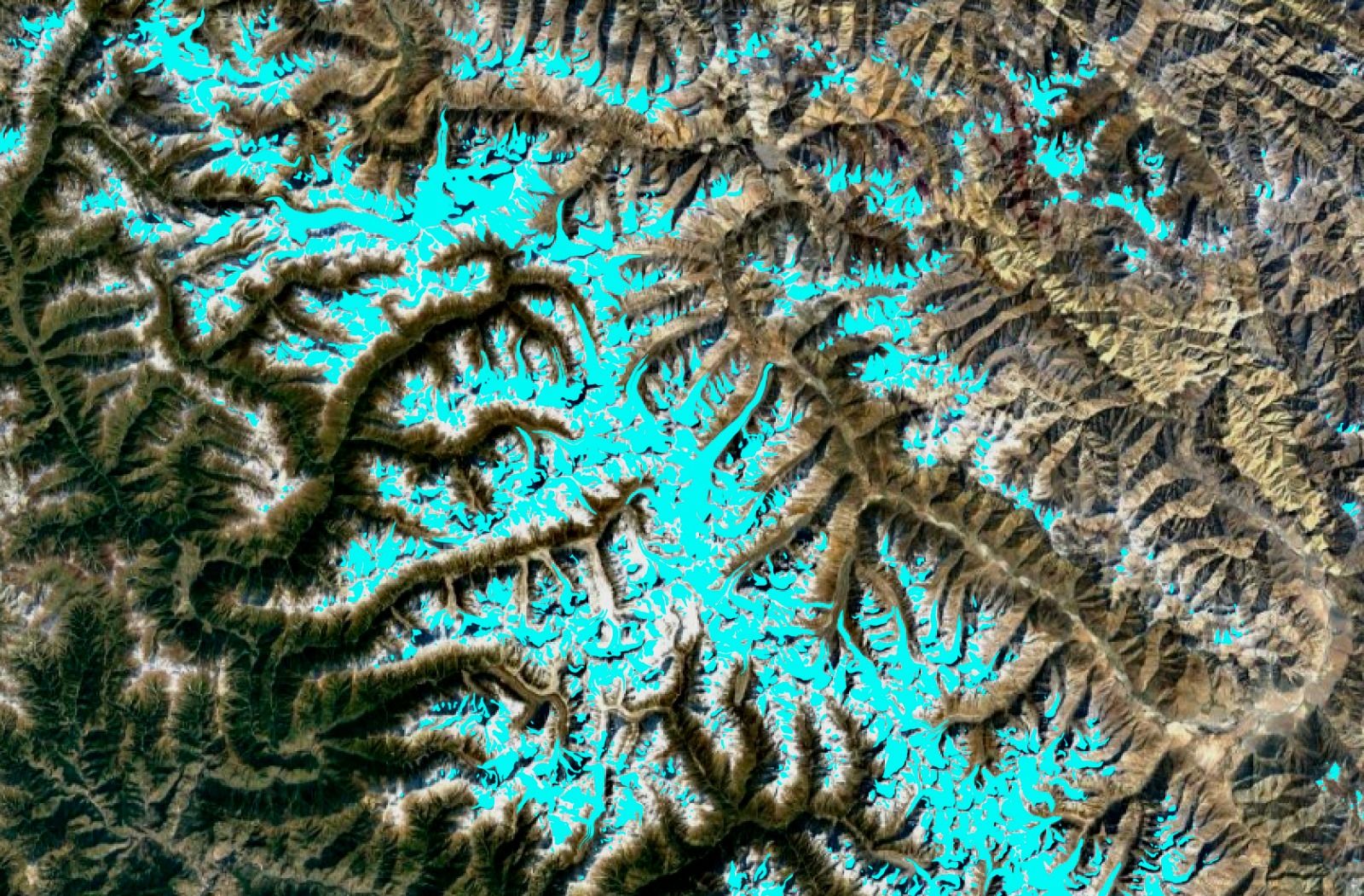
By Claire Gorman
The present era of global climate emergency invites consideration of the “public interest” in environmental terms. Refracted through atmospheric aerosols or acidifying seawater, the environmental public interest may appear in the form of a universal human imperative: to preserve the only planet our species has ever known. However, upon any close inspection this mirage fragments into a staggering multitude of local, cultural, and generational interests, quickly disintegrating from the planetary into the particular. As climate change threatens some groups with inundation and others with drought, some with severe storms and others with runaway wildfires, it produces a profusion of environmental publics, each associated with one of an overlapping multitude of interests. Viewed from an environmental perspective, the public interest is a fractal network of contingencies and incentives, producing a notion of the “environmental public” that has many perspectives and many voices.
In recent years, a new voice has joined the cacophony, verbalizing in an uncanny register that obscures and twists both the planetary and the particular. Artificial Intelligence (AI) has progressed at a rapid pace, providing synthetic environmental data, calculated forecasts of the future, and now automatically generated prose to the conversations that shape environmental action. AI models ingest large amounts of information and make predictions based on the statistical trends they learn from that data, fabricating a perspective that appears at once universal, in its use of seemingly objective large datasets; and individual, in its representation of findings in terms of authoritative most-likely scenarios. While these models have no “interest” of their own and will not be given such ethical standing in this column, they are becoming increasingly embedded as informants of the environmental public.
In particular, the latest wave of Large Language Models (LLMs) lends a new rhetoric to the voice of AI in environmental arenas. The applications of LLMs to environmental policy and planning are extensive: these models can accelerate the drafting and summarization of documents, assist surprisingly complex programming tasks, and rapidly consolidate relevant source material for research conducted by humans. However, the elusiveness of truth within their internal representations poses a stumbling block to the utilization of LLMs in environmental fields, where facts are already disputed and the stakes of research and advocacy are high.
LLMs generate text stochastically, using massive datasets of human-authored prose to learn patterns that allow them to generate their own. It is commonly proclaimed that these models have trained on “the entire internet,” making them uncritical imitators of a public forum where truth coexists with misinformation. With regard to climate in particular, online forums are filled not only with the discord of a fragmentary environmental public, but also rife with corporate-sponsored content disguised as journalism. For example, when fossil fuel companies like ExxonMobil spent decades publishing paid, editorial-style advertisements denying climate change in forums as respected as the New York Times, they poisoned the internet with text that very few humans, let alone LLMs, can identify as biased.
The task of directing Large Language Models towards climate projects strikes at some of the unique problems surrounding the environmental public interest. While a public interest implies a constituency with some perspective, text generated by AI comes from no perspective at all, raising questions of how information with no author can be interpreted in a field where authorship is a primary signifier of legitimacy. Nonetheless, not all kinds of textual information have equal standing in relation to the truth; here, a new environmental media literacy must take on the difference between AI-assisted computer programming and artificially generated firsthand accounts. Sorting through the relationships among AI, environment, and facts will be a key epistemic issue as the proliferation of AI tools coincides with our escalating climate crisis.
To that end, this column intends to critically explore LLMs and adjacent tools as environmental technologies. It will assess their risks and best uses in relation to environmental policy and planning, providing observations through research, expert interviews, and technical explanation that might advise their adoption by environmental professionals as well as their interested neighbors. The agenda of this column is set with recognition of the yet undetermined power of these new intelligences, and with faith that they can be used for good by an informed environmental public.
Claire Gorman is a dual Masters student at MIT pursuing degrees in Environmental Planning and Computer Science. Her research interests include deep learning-based computer vision methods, remote sensing for ecological sustainability, and design as a mediator between science and society. Her bachelor’s degree is in Computer Science and Architecture, from Yale University.